Enhancing Data Anonymization: A Semantic K-Anonymity Framework with ML and NLP Integration
Keywords:
Anonymization, Machine Learning (ML), Natural Language Processing (NLP), Privacy, Semantic K-AnonymityAbstract
This study introduces an innovative framework that enhances data anonymization by integrating semantic k-anonymity with advancements in machine learning (ML) and natural language processing (NLP). Addressing the critical need for robust privacy protection mechanisms, this research responds to the escalating sophistication of deanonymization techniques, which threaten personal data privacy. Through a comprehensive evaluation, including the utilization of the Fβ score, our framework demonstrates superior capability in safeguarding personal data privacy and preserving data utility for analysis. The adaptability to various Θ thresholds and the framework’s proficiency in retaining non-sensitive queries underscore its potential as a groundbreaking solution in data anonymization. This paper not only highlights the framework’s theoretical and practical contributions to digital privacy but also charts a course for future research aimed at navigating the evolving landscape of data protection in an increasingly digital world.
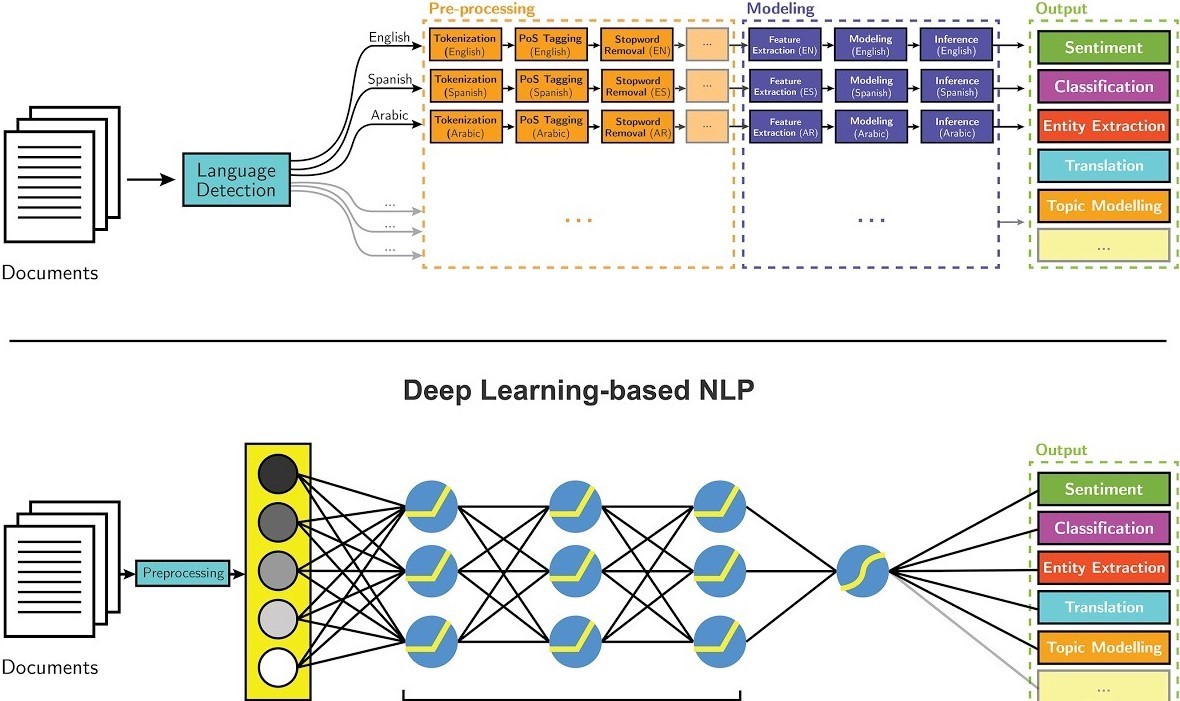