Adopting Generative AI in Digital Marketing Campaigns: An Empirical Study of Drivers and Barriers
Keywords:
Adoption, Barriers, Content Personalization, Digital Marketing, Drivers, Efficiency and Scalability, Generative AI, Machine Learning ModelsAbstract
Digital marketing, being a results-driven field, necessitates a clear understanding of the tangible benefits and potential drawbacks of integrating new technologies. Generative AI promises efficiency, scalability, and personalization in content creation, which are key factors in successful digital marketing campaigns. However, concerns such as the complexity of AI systems, the requirement for human oversight, the potential for reduced creativity, and challenges in integration with existing marketing tools pose significant barriers. This study aims to explore the factors influencing the adoption of Generative AI in digital marketing campaigns. A questionnaire survey was conducted among 411 professionals across three roles: Digital Marketing Managers, Content Strategists or Managers, and Social Media Managers. The primary goal was to understand the drivers and barriers affecting the adoption of Generative AI, categorized into three adoption classes: Not Adopted, Undecided, and Adopted. The methodology involved both traditional statistical models (Ordered Choice and Probabilistic models) and machine learning approaches (Decision Tree, Random Forest, SVM, and KNN). The findings from the Ordered Choice model indicate significant positive coefficients for variables like Efficiency and Scalability, Content Generation and Personalization, Cost-effectiveness, Data-Driven Insights and Automated Optimization, and Chatbot Integration and Innovative Campaigns. In contrast, negative coefficients were observed for Complexity and Human Editing Requirement, Risk and Uncertainty, Integration Challenges and Compatibility, Limited Creativity and Idea Generation, and Limited Knowledge of Recent Events. The Probabilistic model echoed similar trends. The machine learning models demonstrated varying degrees of accuracy in predicting adoption classes, with SVM showing the highest accuracy at 83%. The Random Forest and Decision Tree models highlighted the importance of variables such as Efficiency and Scalability, Content Generation and Personalization, and Cost-effectiveness. The study reveals that while efficiency, scalability, and the potential for personalized content generation drive the adoption of Generative AI in digital marketing, concerns about complexity, creativity limitations, and knowledge gaps pose significant barriers.
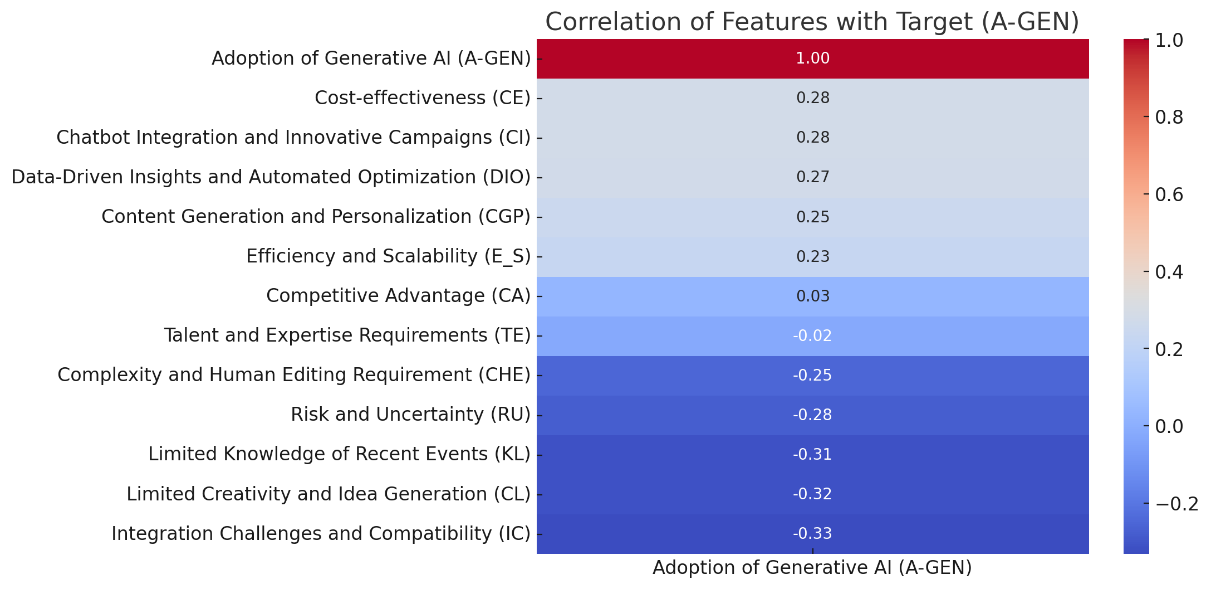