Machine Learning for Predicting Wine Quality and its Key Determinants Based on Physicochemical Properties
Keywords:
Machine learning algorithms, Physicochemical Properties, Quality Assessment, Sensory Evaluation, Wine QualityAbstract
Traditional methods of assessing wine, which lean heavily on human experts for sensory evaluation, are not only time-consuming and expensive but also fraught with inconsistencies due to the inherent subjectivity of taste tests. These expert opinions can vary widely, as taste perception differs greatly among individuals, leading to a lack of standardization in the evaluation process. In this context, there's a growing need for a more objective, efficient, and cost-effective method to assess wine quality. Machine learning offers a promising solution by automating the quality assessment process, leveraging data from physicochemical tests. The aim of this study is to develop and validate a machine learning model for accurately predicting wine quality, based on its physicochemical properties. It seeks to identify key factors influencing wine quality and compare the effectiveness of different machine learning algorithms. Eight different models were applied on the wine quality dataset, and SHAP (SHapley Additive exPlanations) was used to identify the key factors of wine quality. All of the algorithms showed high accuracy scores. The analysis of wine quality using machine learning models highlighted alcohol content as the most influential factor for higher quality, while volatile acidity was negatively correlated with quality. SHAP values identified sulphates, alcohol, and volatile acidity as key determinants of wine quality. After hyperparameter optimization, the best model trial achieved 91.15% accuracy with fine-tuned parameters, although precision and F1-Scores for certain classes slightly decreased. The overall accuracy of the model also experienced a marginal reduction. Despite these changes, SHAP values continued to indicate the importance of sulphates, alcohol, and volatile acidity in the model's decision-making, highlighting their significant role in defining wine quality. This approach could be beneficial for wine distributors, and consumers by offering an objective and scalable method for evaluating wine quality. Additionally, understanding how various chemical components influence quality perceptions could be useful for wine producers aiming to improve their products.
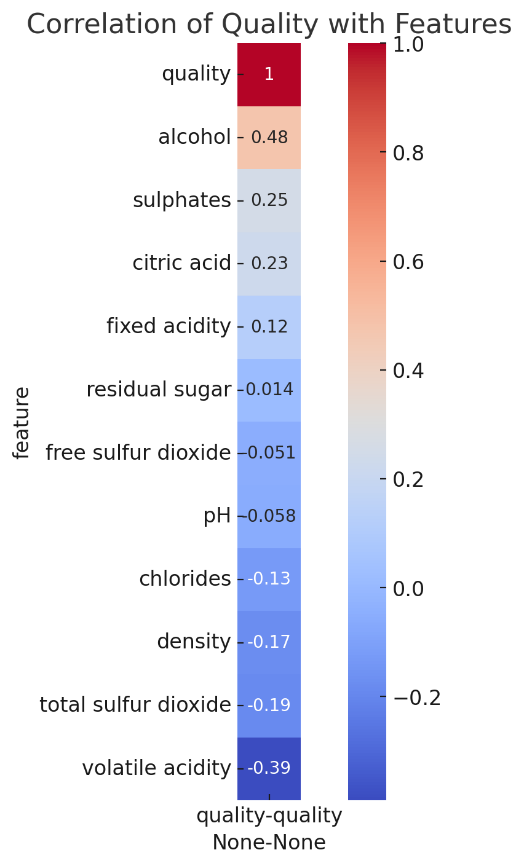