Brain Tumor Segmentation Using Deep Transfer Learning Models on The Cancer Genome Atlas (TCGA) Dataset
Keywords:
Brain Tumor Segmentation, TCGA Dataset, Medical Image Processing, EfficientNet, NasNet, DenseNet, InceptionV3Abstract
Brain tumors stand as one of the primary health concerns internationally, leading to a significant number of fatalities due to the aggressive proliferation of tumor cells. Immediate analysis and automated detection are being used in mitigating the mortality rate. Traditional techniques of brain tumor segmentation and classification, especially when solely reliant on standard medical image processing, exhibit inherent complexities. Medical records suggest that manual classifications, even with human assistance, often fall short in their accuracy due to the distinctions between tumors and healthy tissues. Recently, deep learning methodologies have demonstrated their efficacy in various computer vision applications, including image classification. These deep learning techniques offer the advantage of designing models that can learn and make decisions from given sample data. Transfer learning methods are believed to adjust for inconsistencies in MR images resulting from varying imaging protocols or scanners, allowing for the reuse of deep learning models for related applications. For this study, a dataset of brain MR images, with accompanying manual FLAIR abnormality segmentation masks, was used. These images, sourced from The Cancer Imaging Archive (TCIA), represent 112 patients from The Cancer Genome Atlas (TCGA) lower-grade glioma collection. The research employed four deep learning architectures: EfficientNet, NasNet, DenseNet, and InceptionV3. Our findings indicated that EfficientNet exhibited the highest accuracy in training (0.83) and validation (0.82), followed by NasNet, DenseNet, and InceptionV3. Furthermore, EfficientNet scored the highest in performance metrices, AUC, Sensitivity, Specificity, F1-Measure, and mAP.
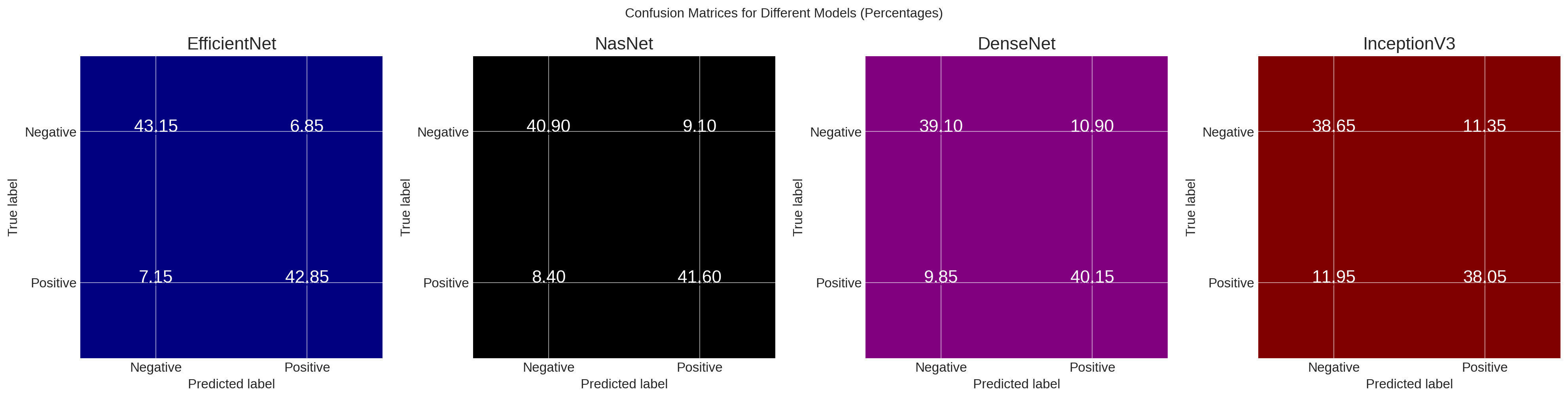