An AI and ML-Enabled Framework for Proactive Risk Mitigation and Resilience Optimization in Global Supply Chains During National Emergencies
Keywords:
Adaptive Response Engineering, Artificial Intelligence, Blockchain Traceability, Emergency Threat Detection, Resilient Supply Chains, Simulation Modeling, Supply Chain TransparencyAbstract
National emergencies in the form of pandemics, natural disasters, cyberattacks, and geopolitical conflicts pose a critical threat to the stability of global supply chains, often causing critical shortages and logistical bottlenecks. This paper presents a proactive focused framework that uses Artificial Intelligence and Machine Learning to make supply chains more resilient and ensure business continuity during such crises. The framework incorporates four major building blocks that form the basis of an integrated approach: Emergency Threat Detection, Dynamic Impact Simulation, Adaptive Response Engineering, and Resilience Monitoring and Automation. Within this framework, the integration of data from IoT devices, satellite imagery, social media feeds, and government alerts enables real-time analytics to help identify emerging risks and forecast cascading effects across tiers in the supply chain. It achieves this by optimizing responses with advanced simulations, from reinforcement learning-based scenario modeling to graph neural networks for disruption propagation analysis. Further, ML-driven algorithms reconfigure the logistics and prioritize resource allocation in such a way that the essentials reach the affected regions as soon as possible. In prolonged emergencies, monitoring and blockchain-backed traceability enable supply chain transparency that empowers the quick identification of secondary disruptions and quality control issues. This would include phased implementation, building a robust data infrastructure, integrating AI/ML pipelines, collaboration across functions, and iterative refinements through feedback loops. This study argues that using such proactive framework, organizations can effectively reduce the risk factors beforehand, minimize operational downtime, and strengthen public trust as supply chains become adaptive, reliable networks during national emergencies.
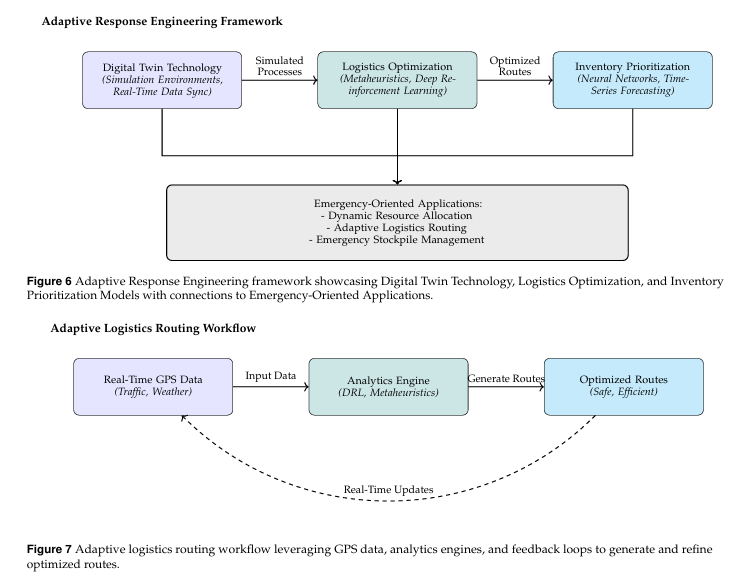