Assessing the Impact of Data Quality on Predictive Analytics in Healthcare: Strategies, Tools, and Techniques for Ensuring Accuracy, Completeness, and Timeliness in Electronic Health Records
Keywords:
Data cleaning, data governance, data quality, Electronic Health Records, predictive analytics, real-time validation, timelinessAbstract
The performance of predictive analytics in healthcare is fundamentally dependent on the quality of the data ingested by predictive models. This paper provides an analysis of how variations in data quality—specifically focusing on accuracy, completeness, and timeliness—affect the efficacy and reliability of predictive models in healthcare. Using Electronic Health Records (EHRs) as the primary data source, this study investigates the influence of data degradation on the precision and utility of predictive outputs in clinical decision support systems (CDSS), patient outcome forecasting, and resource optimization. The research shows the negative effects of data inaccuracies, missing entries, and delayed data entry on model outcomes which can lead to suboptimal or hazardous clinical decisions. Strategies for improving data quality through data governance frameworks, standardization protocols, and real-time validation techniques are examined. Machine learning (ML)-based anomaly detection systems, AI-driven data cleaning algorithms, and EHR-integrated validation processes, are assessed for their ability to improve data quality at scale. This study also proposes automated solutions for monitoring and error correction to ensure data integrity and timeliness in dynamic healthcare environments for optimizing predictive analytics performance in clinical and operational settings.
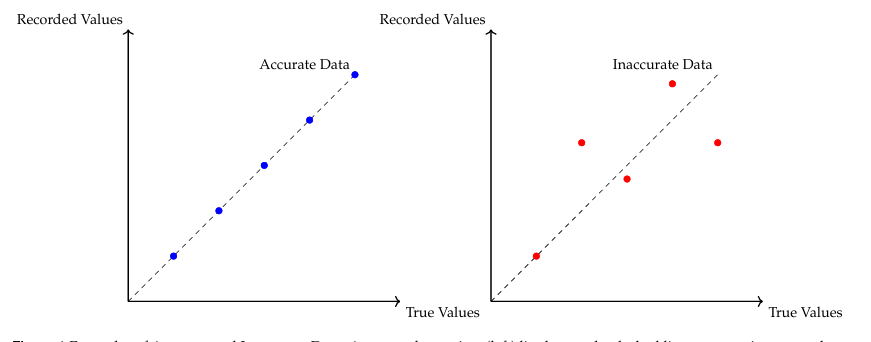