Integrating Robotic Process Automation and Machine Learning in Data Lakes for Automated Model Deployment, Retraining, and Data-Driven Decision Making
Keywords:
Automation, Data Lakes, Machine Learning, Model Deployment, Robotic Process Automation, Scalability, System InteroperabilityAbstract
The integration of Robotic Process Automation (RPA) and Machine Learning (ML) within data lakes is a progressive strategy to improve automated model deployment, retraining, and data-driven decision making. Data lakes serve as centralized repositories that allow the storage of structured, semi-structured, and unstructured data at scale, providing a foundation for advanced analytics. The convergence of RPA and ML facilitates the automation of repetitive tasks, accelerates data processing, and refines model accuracy through continuous learning. This paper discusses the methodologies for integrating RPA and ML in data lakes, addressing the infrastructure, technologies, and workflows involved. The benefits of this integration, such as improved efficiency, cost savings, and enhanced decision-making capabilities are also discussed. The paper also explore the challenges and solutions associated with implementing this hybrid approach, including data governance, system interoperability, and the scalability of machine learning models. Through examining current industry applications, the study highlights best practices and strategic considerations for organizations aiming to use this integration for competitive advantage. The paper concludes by identifying future trends and research directions in the domain of RPA, ML, and data lakes, emphasizing the transformative impact on various sectors, including finance, healthcare, and manufacturing.
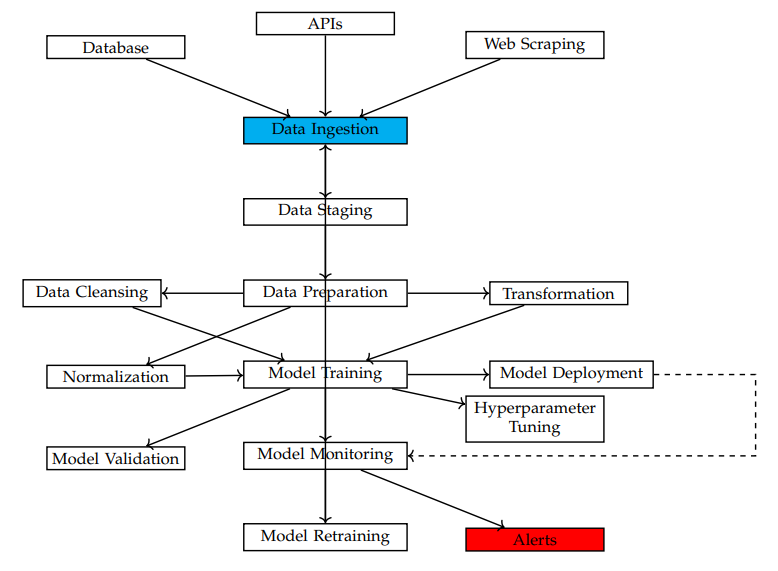