Machine Learning and Big Data Analytics for Cybersecurity Threat Detection: A Holistic Review of Techniques and Case Studies
Keywords:
Machine Learning, Big Data Analytics, Cybersecurity, Threat Detection, Holistic Approach, Data Processing, Anomaly DetectionAbstract
In the ever-evolving landscape of cybersecurity, the effective detection of threats is paramount to safeguarding digital assets and privacy. This research article presents a holistic review of the integration of machine learning and big data analytics in cybersecurity, shedding light on their pivotal role in identifying and mitigating cyber threats. The research elucidates the significance of these technologies in enhancing security measures and underscores the imperative for a comprehensive approach to threat detection. Machine learning techniques are examined in depth, revealing their capacity to process vast datasets and rapidly pinpoint anomalies and potential threats. Case studies demonstrate their practical applications, including the detection of malware, phishing attempts, and network traffic anomalies, validating their utility in real-world scenarios. Concurrently, big data analytics is explored as a vital component in managing and deriving actionable insights from the massive volumes of data generated in the digital age. Through the utilization of specialized tools, big data analytics enables organizations to uncover hidden threats and act proactively to minimize risks. Case studies exemplify how big data analytics identifies patterns and correlations, enabling timely responses to evolving threats. The synergy of machine learning and big data analytics is emphasized as the cornerstone of a holistic approach to cybersecurity. By combining machine learning's adaptability and big data analytics' data processing capabilities, organizations gain a comprehensive, real-time view of their security posture. This approach ensures that historical data, real-time information, and predictive analytics converge to form a formidable defense against cyber threats. Ethical considerations are also integrated into the approach, addressing privacy concerns associated with data collection and processing. This research article concludes by highlighting the significance of machine learning and big data analytics in contemporary cybersecurity and the necessity for a holistic and adaptive security posture. It encourages ongoing investment in research and development, proactive knowledge updates, and the upholding of privacy rights in the ongoing battle against cybercrime.
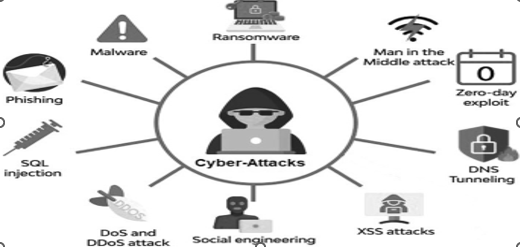